About the factsheet
Pharmaceutical organizations have a safety data problem—there is a growing volume of safety events in increasingly varied formats. This is leading to unsustainable increases in the costs of traditional safety operations.
Overview
Natural language processing (NLP) text mining can optimize safety platforms and lower clinical development costs. NLP transforms unstructured text into structured data that can be rapidly analyzed or visualized. This capability can be applied for safety case processing, medical coding (e.g. to the Medical Dictionary for Regulatory Activities, or MedDRA), publication search for potential adverse event (AE) situations and medical review of AEs, and at every stage through the safety life cycle of a drug.
Drug safety is of critical importance at all stages in drug discovery, development and delivery. Across the whole span, safety-relevant data is being both generated and sought from unstructured text. This includes safety case reports, emails, call center feeds, scientific literature, internal safety documents, social media and more. Finding and extracting the AE itself, plus the relevant context around it, is becoming increasingly burdensome for safety assessment and pharmacovigilance teams using traditional manual processes. However, new artificial intelligence approaches such as NLP are changing the safety vigilance technology landscape, and pharma companies are looking at these advances to optimize their safety platforms and lower clinical development costs.
Linguamatics NLP can address some of these challenges by efficiently and comprehensively extracting AE data from the mass of unstructured text sources. NLP can search and extract AEs, symptoms and indications, patient history, drug names, dosages, and other relevant context from unstructured documents. These capabilities are applied within pharmacovigilance, for example for MedDRA coding of AEs in safety case processing, or for understanding the context of a particular potential safety signal in safety assessment.
Key capabilities
- Safety-related ontologies, to boost recall. Linguamatics biomedical terminologies enable identification, extraction and normalization of over a million concepts, covering a wide variety of life science domains: Diseases, genes, proteins, biomarkers, gene variants and mutations, phenotypes, drugs, AEs, biological processes, organs, tissues and cells.
- The ChemAxon add-on identifies known and novel chemical structures within documents by name, structure, substructure or similarity. In addition, pattern ontologies identify numerical data such as dosages, dates, numerics and units of measurement.
- Linguistic components: These components make search more precise by looking for linguistic relationships, for example the relationships between concepts (e.g. search for compounds causing disease rather than treating disease; see Figure 1), or using context to differentiate between an AE and negative context, family history or patient indication (see Figure 2). Document regions can also be used to make search more precise, including accessing textual data within embedded tables.
Figure 1: Figure 1: Results from search in MEDLINE abstracts showing relationships between a drug, nivolumab, with different observations
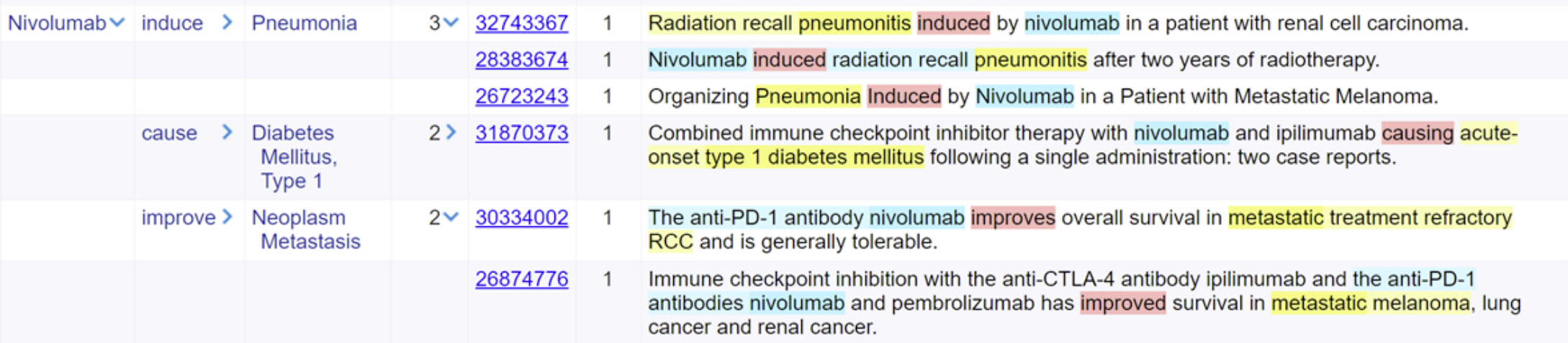
In these documents, nivolumab is related to pneumonia and diabetes by causal relations, and to metastatic cancer with a “treats” type relationship.
Use cases of NLP within drug development
The following use cases from CSL Behring, AstraZenenca, Lilly and Agios Pharmaceuticals exemplify the successful application of the NLP platform to extract, detect and monitor AEs and safety signals.
CSL BEHRING: NLP TO SUPPORT MEDICAL CODING IN POST-MARKET SAFETY CASE PROCESSING
Once a drug is on the market, pharma companies need to screen huge volumes of reports for potential AEs from patients in the real world. Within pharmacovigilance workflows, reports are received from many sources—call center feeds, emails, regulatory AE reports and more. These are often in everyday language, and so have to be coded into a standardized format to allow database processing. For the AE, indication, medical history, etc., MedDRA must be used. Most of the coding is manual and time consuming. Only when the verbatim exactly matches a MedDRA term is coding automatic. For example, a verbatim might read: “I really got ill the other day, had a horrible headache and couldn’t sleep for two days.” “Headache” has an exact match in MedDRA, so can be auto-encoded, but “couldn’t sleep” is not a MedDRA term and so has to be manually coded to “sleeplessness.” worked with CSL Behring to develop an NLP workflow that doubled the level of auto-coding (from only 30% of AEs to over 60%). Use of NLP improved coding consistency, and can reduce risk for case processing and medical evaluation.
Figure 2: Biomedical observations are often found in free text that are not AEs
Linguistic patterns can filter out inappropriate contexts.
NLP TO SUPPORT CONTEXTUALIZATION OF SAFETY SIGNALS
AstraZeneca wanted to understand the potential of a particular safety signal, neutropenia, to translate from preclinical to clinical. Building an understanding of the landscape around drugs causing neutropenia would enable them to build predictive models from preclinical to clinical. However, data on drugs reported to cause neutropenia in humans and model animal species are buried in scientific literature and other textual sources. They used NLP to mine scientific abstracts and clinical trial reports to find evidence of drugs causing the condition, specifically searching for drug and condition in phrase with a suitable verb (looking for causality).
Linguamatics NLP enabled them to build queries that looked for modifiers (e.g. drug-induced, transitory, severe, prolonged) and also species information (e.g. rat, mouse, dog). This rich landscape of structured data was used to feed predictive models from preclinical to clinical, and enabled the application of models to drugs at preclinical stages, before clinical trials began.
NLP TO ENHANCE THE SEARCHABILITY OF INTERNAL PRECLINICAL TOXICOLOGY SAFETY REPORTS
There is a wealth of valuable information in legacy preclinical safety reports. Locked away in these historical data are answers to questions such as: Has this organ toxicity been seen before? In what species, and with what chemistry? Many pharma organizations use document repositories to store their preclinical tox studies, but the search functionality of many of these document management systems is limited, hindering access. At Lilly, they use Linguamatics NLP to enable rapid effective searches over the toxicology reports. The intuitive NLP web portal allows scientists who aren’t experts in NLP to run effective searches and pull back the data and documents of interest, for downstream review and analysis.
NLP FOR CLINICAL SAFETY AT AGIOS PHARMACEUTICALS
Agios Pharmaceuticals says that it “uses [Linguamatics] NLP to get decision support as fast and as comprehensively as possible.” They apply NLP in clinical safety workflows to mine AE reports and assist with initial coding of reported events and WHO drugs. In a specific case, Agios explored the risk of a rare, yet potentially life-threatening AE, Differentiation Syndrome, in patients on a clinical trial for Agios’ IDH-1-inhibitor AG120. Linguamatics NLP was used to extract key information from Serious Adverse Event Report Forms, and the extracted data was visualized as networks in Cytoscape. This enabled clinicians to explore the patterns of symptoms between patients and, critically, to identify those at riskof the potentially fatal AE.
Digital transformation for safety innovations
Safety is assessed throughout the life cycle of a drug, from bench to bedside. The ultimate test is after the drug has been approved and used in clinical settings with many thousands of patients, across broader indications, and combined with other drugs. There are always risks, but the more relevant data that can be extracted, analyzed and transformed into actionable insights and information, the greater the likelihood of lowering that risk.