Drive population health analytics and health equity
Why address social determinants of health?
To understand the real cost of food insecurity, a validated micro-simulation model — the cardiovascular disease (CVD) policy model for risk, events, detection, interventions, costs and trends (PREDICT) — was initiated to project the health and cost outcomes, at both an individual and population level, relating to risk factors and/or treatment status for CVD. This model found that increasing intake of healthy fruits and vegetables by 30% was estimated to prevent 1.93 million CVD events, gain 4.64 million quality-adjusted life years and save $39.7 billion in formal healthcare costs.1
Core Determinants of Health
Core Determinants of Health
- Living conditions
- Limited English
- Social isolation
- Stress
- Substance abuse
- Transportation issues
- Victim of crime and violence
- Weight range category
- Physical activity
- Depression
- Employment status
- Financial issues
- Ambulatory issues
- Food insecurity
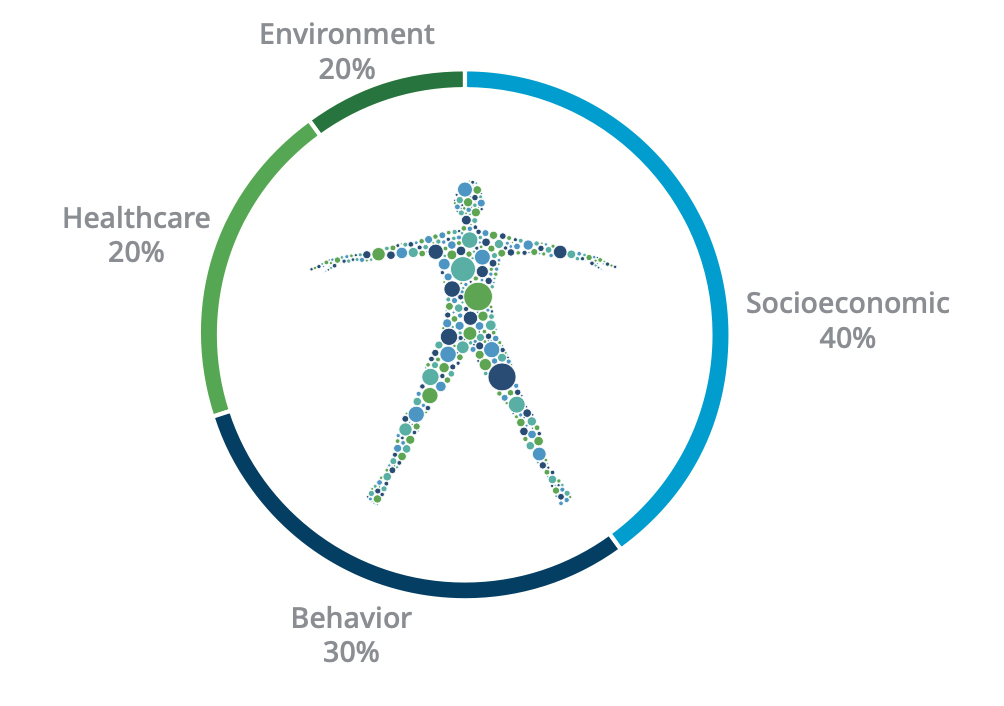
Source: University of Wisconsin, Population Health Institute
Social isolation comes at a hefty cost to individuals and society in general. Social isolation is connected to roughly $6.7 billion in additional healthcare costs annually among Medicare beneficiaries and contributes to poor health outcomes, including a 50% increased risk of early mortality.2
Surfacing SDOH data at the individual patient level
There is an increased focus on the social determinants of health (SDOH) that has led to organizations trying to better understand how these impact patient outcomes. These complex and nuanced features are poorly defined and rarely included in structured data. There is effort directed at better capture of this information within structured data within the electronic medical record (EMR) — but asking clinicians, who are already fighting burnout, to fill in another form has not yielded an uptick in SDOH screening data.
Many organizations assume this data does not exist within their own systems and are instead buying aggregate zip code or census data to infer insights about those most at risk. However, this data is not patient-specific and may not include key data related to SDOH measures such as:
- Does the patient have access to transportation?
- Does the patient live alone?
- Can the patient ambulate?
But SDOH data does exist in the medical record. It is captured every day by trained medical professionals, taking detailed clinical histories from their patients, just as they were trained to do at medical school and have always done. The data are in admission notes, care management systems, and discharge summary and nursing notes. The problem is surfacing this information in a repeatable and reliable way. Fortunately, with artificial intelligence (AI) technologies such as Linguamatics NLP (natural language processing), data can be found and normalized for use in health equity initiatives.
SDOH information extracted and ready to analyze
With Linguamatics NLP, SDOH information is normalized to 14 topics, with different numbers of classes per topic. The Linguamatics NLP engine identifies misspellings, synonyms, context, negations, etc to ensure that information in the medical record is extracted as it was meant by the clinician who entered it.
In addition, the output includes suggested SNOMED and ICD-10 codes along with their descriptions for each topic.
Tailored and accurate NLP algorithms are available out- of-the-box to get you to the SDOH information quickly. Alternatively, they can be modified to capture more information of interest.
Contact us for more information about how your organization can repeatably harness NLP to extract SDOH information and drive health equity.
Value of NLP within safety vigilance
- NLP can be used to screen case reports, social media, abstract and full text literature, and other textual unstructured or semi-structured sources for drug–AE associations.
- Queries can be built to identify context (patient, reporter, dosage, species, other key features).
- NLP can provide access to external content valuable for safety contextualization (e.g. MEDLINE abstracts, PubMed Central open-access full-text papers, FDA Drug Labels, ClinicalTrials.gov, FAERS).
- Linguamatics NLP can be integrated into workflows for automation, AE alerts, or can used interactively to search for specific safety-related information.
- Linguamatics NLP can be applied at any point where literature review is needed, for single case handling, aggregate reporting, signal identification, summary reports and more.